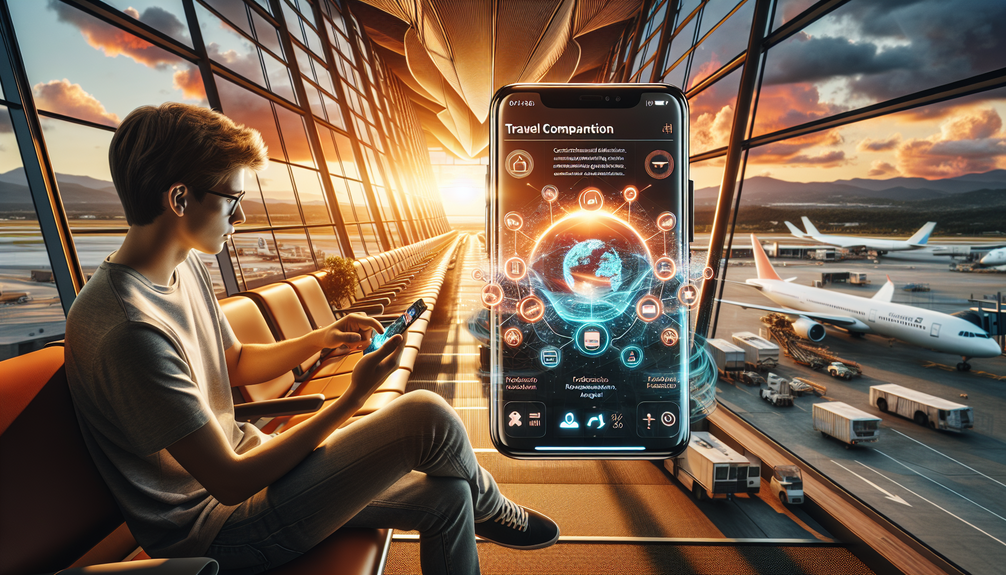
AI News
27 Jan 2025
Read 6 min
How Human Feedback Revolutionized Travel AI with Near-Perfect Accuracy
Smarter travel planning with AI tools enhanced by human feedback means personalized trips made easy
Introduction to AI Travel Tools
Artificial intelligence has changed how people plan trips. Travel tools that use AI can help travelers book flights, find hotels, and create itineraries. But AI tools were not always accurate in understanding user needs. Travelers often gave up on AI-based tools because the recommendations felt impersonal or missed key details.
Today, AI travel systems are smarter. They process information better and respond like a real human assistant would. This improvement became possible through reinforcement learning with human feedback (RLHF). This method is reshaping travel tools by adding a human layer to complex machine algorithms.
What Is Reinforcement Learning with Human Feedback?
Reinforcement learning is a type of machine learning. In this process, AI learns by receiving rewards or punishments for actions. The AI aims to maximize rewards over time by adapting its behavior.
In the RLHF model, human feedback acts as an additional form of guidance. Instead of only using numerical rewards, human trainers rate AI responses for quality and relevance. This creates a training loop where humans directly influence AI behavior. As a result, the AI becomes more accurate and user-friendly in real-world applications.
Why RLHF Matters for Travel AI
AI in travel can often misunderstand user preferences. For example, some users prioritize cost, while others care about comfort or speed. Traditional models fail to deliver custom responses because they rely solely on predefined algorithms.
RLHF fixes this issue because it introduces human understanding into AI decision-making. Here are some key reasons why this makes a difference:
- Creates more personalized itineraries by understanding user priorities.
- Improves AI’s ability to handle specific travel requests, like changes in plans.
- Enhances user satisfaction by resolving ambiguity in prompts.
The shift to RLHF has made AI-driven travel tools more practical and reliable.
Klopp and Matador: Leaders in Travel AI
Two startups, Klopp and Matador, are using RLHF to improve travel planning tools. Both companies focus on helping users book more efficiently, avoid common travel problems, and explore new destinations. Their systems leverage large language models (LLMs) such as OpenAI’s GPT framework but are trained further using human feedback.
How Klopp Improves User Experiences
Klopp has developed a travel assistant designed for accuracy and user comfort. It uses RLHF to deliver smart suggestions that match individual preferences. For instance:
- It can prioritize affordable options for budget travelers.
- It identifies local attractions based on user input.
- It adapts to last-minute changes, such as rebooking flights or accommodations.
Klopp has gained attention for reducing the time needed to plan a trip. The tool’s interface is user-friendly, making it accessible to people without tech experience.
Matador’s Focus on Exploration
Matador’s travel system emphasizes outdoor adventures and unique experiences. It uses RLHF to provide personalized travel guides. Matador’s AI also focuses on analyzing user reviews, ratings, and feedback to improve suggestions over time.
By integrating RLHF, Matador creates tools that ensure travelers make informed decisions. This is especially useful for those exploring remote destinations or seeking eco-friendly vacation ideas.
Benefits of RLHF-Powered Travel AI
The use of RLHF offers significant advantages to travelers. By blending machine precision with human understanding, travel tools now provide more effective solutions.
Increased Accuracy
Traditional AI struggled with accuracy in understanding vague or complex queries. RLHF bridges this gap by refining responses with human oversight. Travelers now receive better recommendations, such as optimal transportation routes or alternate plans during disruptions.
Improved Context Awareness
Travelers often use casual language when asking for information. RLHF helps AI tools interpret informal queries better. For example, if a user says, “I want a cheap flight but hate long layovers,” the AI can deliver accurate results that match the request.
Time-Saving Features
By leveraging RLHF, travel systems can detect patterns and preferences quickly. This reduces time spent on planning and research. Travelers can book complete trips from flights to activities in minutes rather than hours.
Better Post-Booking Support
Modern travel AI tools can assist users even after their bookings are complete. They send reminders, provide directions, and offer alternate suggestions if plans change. RLHF ensures that these services are not only helpful but also feel more considerate.
Challenges to Implementation
While RLHF is transforming the travel sector, its integration is not without hurdles. Companies face certain challenges as they work to refine these systems further.
- High costs: Training models with human feedback requires significant resources.
- Scalability: Scaling RLHF systems to handle millions of users remains a technical obstacle.
- Bias risks: Human trainers may introduce subjective biases during the training process.
Despite these difficulties, Klopp and Matador, among others, are investing heavily in making RLHF scalable and unbiased.
The Future of Travel AI
Reinforcement learning with human feedback is shaping the future of travel planning. As companies like Klopp and Matador continue innovating, more users are likely to rely on AI tools. Key features such as real-time adaptability, increased personalization, and multilingual support are on the horizon.
In the coming years, travel AI tools powered by RLHF may evolve into one-stop solutions for every travel need. From planning to booking to local guidance, they promise to make traveling smoother, faster, and more enjoyable for everyone.
(Source: https://news.crunchbase.com/ai/reinforcement-learning-human-feedback-travel-tool-klopp-matador/)
For more news: Click Here
Contents