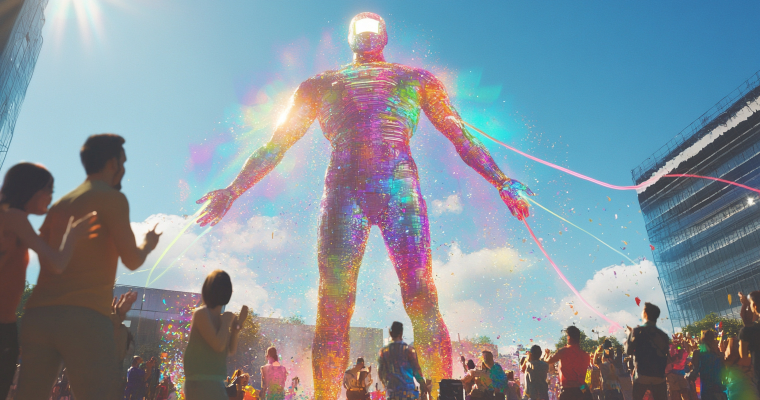
Prompts
14 Apr 2025
Read 20 min
Mastering Prompts: Insights from Google Viral Paper on Prompt-Engineering
Prompt-engineering yields better AI results using concise instructions.
Abstract and Author Background
Lee Boonstra is an engineer at Google. She created a document that explains how to guide large language models (LLMs) using clear prompts. These prompts instruct the model to produce text for specific tasks. Her guide, often called the “Google viral paper on prompt-engineering,” offers step-by-step methods for making AI produce better results. It also shows how to cut down on mistakes and get more accurate answers. This paper has gained attention because it simplifies AI usage for both beginners and experts.
Boonstra’s main focus is to teach ways to interact with LLMs like Google Gemini, GPT, Claude, and more. Her document gives people a way to master prompt design. She also offers tips on setting model parameters, like temperature and token limits. Boonstra shows real examples with code snippets and best practices. She also covers new techniques, such as Chain of Thought (CoT) prompting, self-consistency, and ReAct (Reason and Act). She believes anyone can learn prompt-engineering, no matter their background.
You will see that her instructions are easy to follow. They avoid jargon. They also address how to adapt prompts for each project. This is useful for students, hobbyists, and experienced developers. Boonstra emphasizes short, direct lines for each prompt. She also explains why you should test prompts multiple times. This helps the user discover what works. Her approach shows a clear way to make AI do specific tasks.
Below is a long-form blog post, written in simple English, with a subject-verb-object pattern. It aims to present the key insights of Boonstra’s “Google viral paper on prompt-engineering.” We will explore the techniques that help you instruct AI clearly. We will also discuss how to manage output length and improve accuracy. By the end, you will see how prompt-engineering can transform your AI projects.
Lee Boonstra’s “Google viral paper on prompt-engineering” has inspired a new generation of AI explorers. Her instructions are simple to follow. They show the power of short, direct commands. She also includes advanced ideas for anyone who wants more control over AI. By studying her techniques, you can improve your results with any large language model.
Takeaways:
- You do not need to be an expert in math or coding.
- Simple words work well for AI prompts.
- Different prompt types suit different tasks.
- Model settings, like temperature, affect creativity.
- Testing each prompt is the only way to find the best solution.
Introduction: Why Prompt-Engineering Matters
Are you curious about AI but afraid it might be too hard? You are not alone. Many people worry that they need programming skills or math expertise to use AI. Lee Boonstra’s “Google viral paper on prompt-engineering” shows that anyone can guide an AI model with well-written prompts. In fact, the way you phrase a question can affect whether the AI’s answer is correct or not.
Think about how you talk to a friend. You use short questions or statements to explain what you want. The same method can help you guide AI systems. You do not need fancy words. You just need clarity. That is the main point of prompt-engineering. The goal is to craft simple instructions so the AI produces the results you need.
Boonstra’s guide proves that writing prompts is an ongoing process. You test a prompt, you see what answer you get, then you adjust it. Over time, you gain a sense of what works. You might want shorter answers or more creativity. Changing a few words in your prompt can do all that. This is why the paper has gone “viral.” It demystifies AI communication for users at all levels.
In this blog post, we will detail the main ideas from the paper. We will look at key prompt techniques, how to handle model settings, and how to refine prompts. We will also learn why referencing the author and the source is good for clarity. By the end, you will see how prompt-engineering raises your AI game. You do not need special tools, just a willingness to try.
1. What Is Prompt-Engineering?
Prompt-engineering is the act of giving clear text instructions to an AI model. These prompts guide the AI to produce specific results. For example, if you want an AI to write a short recipe, you say: “Write a recipe for chocolate cake in five steps.” If you want a longer, detailed recipe, you adjust the wording.
Key points to remember:
- Use short phrases in your prompt.
- State your goal clearly (e.g., “Summarize this text in two paragraphs”).
- Add a short example if the AI seems confused.
- Tweak your prompt if the AI’s answer is off.
The “Google viral paper on prompt-engineering” by Lee Boonstra shows many examples. She shows how to create zero-shot prompts, few-shot prompts, or chain-of-thought prompts. These help the AI break down your request and generate better replies.
Zero-shot means you give the AI no examples. You just say what you want. Few-shot means you provide one or more examples. That trains the AI to follow a certain style or format. Chain-of-thought means you invite the AI to think through the problem step by step. Each approach can work for different tasks.
2. Basic Prompt Types
Boonstra’s paper focuses on three basic prompt types: zero-shot, one-shot, and few-shot. We will explain them one by one.
Zero-Shot Prompt
- You give the AI a direct instruction.
- No extra examples.
- Good for simple tasks like “Translate this sentence to French.”
- Quick to write, but less detail.
Example:
“Summarize the news article below in one paragraph.”
One-Shot Prompt
- You give the AI one example.
- It sees how you want the output.
- Good for tasks like “Parse a pizza order.”
- The AI copies the style from your single example.
Example:
“Parse a pizza order into JSON. Example: ‘I want a large pizza with cheese and olives.’ Output:
{“size”: “large”, “type”: “normal”, “toppings”: [“cheese”, “olives”]}
Now parse: ‘I want a small pizza with peppers.’”
Few-Shot Prompt
- You give multiple examples.
- The AI learns from each example’s structure.
- Helpful for more complex tasks.
- Allows you to guide the style of the output.
Example:
“Parse a pizza order into JSON.
Example 1: …
Example 2: …
Now parse: ‘I want a medium pizza with mushrooms and onions.’”
These prompt types form the foundation of prompt-engineering. They help the AI model narrow its guesses. They also show the user how examples change the final text.
3. LLM Output Setup
LLMs like Google Gemini, GPT, or Claude let you adjust certain settings. This includes temperature, top-K, top-P, and output length. Boonstra’s paper explains how each setting affects results:
- Temperature: Controls creativity. Low temperature = stable answers. High temperature = more variety.
- Top-K: Limits the next word to the top K choices.
- Top-P: Limits the next word to a probability threshold.
- Output Length: Sets how many tokens or characters the AI can produce.
Why do these matter? If you want a serious answer, you keep temperature low. If you want creative ideas, raise the temperature. If you only want safe, direct answers, you keep top-K and top-P at stable settings. This ensures the AI does not drift into random territory.
Bullet Points for LLM Output Setup:
- Low temperature: stable, direct answer.
- High temperature: playful, unexpected answer.
- Use top-K or top-P to control how many words are possible.
- Limit output length if you want short or tweet-like replies.
4. System, Role, and Contextual Prompts
Boonstra introduces system, role, and contextual prompts. These let you shape the AI’s behavior more precisely.
- System Prompt: You define the system’s instructions. For example, “You are a helpful teacher. Always use polite language.” Then the AI will adopt that stance.
- Role Prompt: You tell the AI to act as a specific character. For example, “Act as a travel guide. Provide advice for visiting Italy.”
- Contextual Prompt: You give background info. For example, “This text is from a story about ancient Rome. Summarize it.”
These prompt types help the AI adopt a style, tone, or function. It also helps the AI see what context to include or ignore. Boonstra’s “Google viral paper on prompt-engineering” includes code examples for each. She also shows how to combine them. You might have a role prompt plus a system prompt, especially if you want the AI to follow certain rules.
5. Advanced Prompting Techniques
Boonstra does not stop at simple prompts. She dives into advanced methods:
5.1. Chain of Thought (CoT)
Chain of Thought is a method that tells the AI to outline its reasoning before giving the final answer. For example, you say, “Let’s think step by step.” The AI then shows how it solved a math problem. It lists each step of logic. This helps you see if the AI went astray. It also improves the final answer.
5.2. Self-Consistency
Self-consistency means the AI tries several lines of reasoning. Then it picks the answer it finds most common among these lines. It acts like majority voting. If the AI is not sure about a problem, it tries multiple chains of thought. The answer that appears the most is chosen. This can improve accuracy.
5.3. Tree of Thoughts (ToT)
Tree of Thoughts splits the AI’s reasoning into branches. It explores different paths. This helps for tricky tasks. The AI can compare branches and pick the best route. You can see it as a map where each path might lead to a new idea or conclusion.
5.4. ReAct (Reason + Act)
ReAct prompts let the AI gather info by using tools. For example, it might do a search or run some code. It reasons about what to search for, then takes action. This approach is stronger than a basic prompt. It gives the AI direct access to data. It can check facts or gather new material.
Why use advanced techniques?
- You get more accurate answers.
- You see how the AI thinks.
- You can solve tasks that are more difficult or longer.
6. Automatic Prompt Engineering (APE)
Boonstra’s document also touches on a feature called Automatic Prompt Engineering. APE helps you design good prompts without doing it all by hand. You ask the AI to produce multiple prompt ideas. Then you pick the best prompt or refine it.
How it works:
- Write a prompt that asks the AI to generate several prompts.
- Evaluate each prompt’s success.
- Keep or tweak the best prompts.
APE saves time. It also might reveal new approaches you did not consider. Because AI can reword or restructure prompts, you gain fresh perspectives.
7. Prompting for Code
The “Google viral paper on prompt-engineering” also has examples for code generation. This helps developers write scripts or fix bugs faster. You can ask, “Write a Python function that renames files in a folder.” The AI gives you a code snippet.
You can also ask the AI to debug your code. If you show an error, the AI might explain why. It could fix your code or suggest improvements. Make sure you read and test any code you get. AI can make mistakes.
Tips for code prompts:
- Specify the programming language.
- State your function’s goal.
- Request docstrings or comments for clarity.
- Check all generated code for errors.
Boonstra highlights how you can pass a broken script to the AI. The AI can debug it or even rewrite it in a new language. This speeds up development.
8. Best Practices: Short, Clear, and Logge
One main lesson from the document is that clarity rules. If you include filler words, the AI might get confused. If you are too vague, the AI might produce nonsense. If you are too lengthy, the AI might lose track.
Boonstra lists these best practices:
- Provide Examples: One-shot or few-shot. Show the model what you want.
- Design with Simplicity: Use short instructions and simple words.
- Be Specific About Output: If you want JSON, say “Return valid JSON.”
- Use Instructions Over Constraints: State what you want, not only what you do not want.
- Control Max Token Length: If you want a short answer, limit tokens.
- Use Variables: If you have repeated inputs, store them as variables.
- Document Attempts: Keep track of each prompt version and outcome.
These simple guidelines reduce confusion. They also make the AI’s job easier. You are telling it exactly what to do. Boonstra suggests referencing model updates. If the AI changes, test your prompts again. A new version might respond differently.
9. Making AI Work for You
Prompt-engineering is a lifelong practice. It is like training a child or coaching a team. You learn, you test, you refine. As you try new ways to say instructions, you see how the AI reacts. You take note of what works and use it again.
When you adopt these habits, you can solve many problems. Want to summarize a meeting note? Prompt the AI. Need code for data cleaning? Prompt the AI. Looking for a creative birthday greeting? Prompt the AI. By using clear, step-by-step instructions, you shape the AI’s mind. You get results that are often better than random guesses.
10. Common Pitfalls and How to Avoid Them
Boonstra’s “Google viral paper on prompt-engineering” also warns about pitfalls. Here are a few:
- Ambiguity: If your prompt is unclear, the AI can misunderstand.
- Too Many Constraints: A giant “Do not do this, do not do that” can confuse the AI.
- Ignoring Temperature: You might want creative writing but keep temperature too low.
- Failure to Test: You create a prompt once and assume it always works. But the AI might vary.
- Lack of Examples: The AI might guess your format if no examples are provided.
Solution:
- Always read the AI’s first answer.
- Tweak your prompt.
- Use short instructions in plain language.
- Provide examples to guide the style.
11. Why the Paper Went Viral
Lee Boonstra’s guide simplifies a topic many found difficult. She uses real prompts that show a direct cause and effect. Her paper is thorough but not lengthy. She provides code to show practical results. She also includes advanced topics, appealing to experienced users.
People love the idea that you do not need a PhD to talk to an AI. That is why the document is called the “Google viral paper on prompt-engineering.” It cuts through the technical details, offering a direct path to better AI usage. Beginners find it approachable. Experts enjoy the advanced methods. The mix of real examples, clear wording, and easy steps resonates with many.
12. Bringing It All Together
Prompt-engineering is a powerful skill. You learn a few simple rules, then you experiment. The best prompts are direct, short, and tested. Advanced methods like Chain of Thought and ReAct help you tackle bigger tasks. Tools like Automatic Prompt Engineering and system/role prompts add flexibility.
Lee Boonstra’s work shows that all of this is possible with minimal code. You can copy and paste examples. You can adapt them. She suggests you keep track of each attempt and note the outcomes. This way, you can remember what worked last time. That is a simple but effective approach.
As AI moves forward, new LLMs appear. New features might let you feed images or audio. We can apply the same prompt-engineering logic. We say what we want, we check the results, and we refine. The paper’s ideas stay relevant no matter which model we use, from Gemini to GPT to open-source solutions.
Conclusion
Prompt-engineering changes how we use AI. It is not about random questions or guessing. It is about carefully built prompts that lead to clear answers. Whether you are a student, a hobbyist, or a pro developer, you can learn these methods. You can add them to your daily tasks. You can write code, summarize texts, or research facts more quickly.
Lee Boonstra’s “Google viral paper on prompt-engineering” has inspired a new generation of AI explorers. Her instructions are simple to follow. They show the power of short, direct commands. She also includes advanced ideas for anyone who wants more control over AI. By studying her techniques, you can improve your results with any large language model.
Download Document: Click Here
FAQ
- What is “Google’s viral paper on prompt-engineering”? It is a guide by Lee Boonstra from Google. It shows how to write clear prompts for large language models. The methods help beginners and experts use AI more effectively.
- Why do I need simple language for my prompts? Short, direct words reduce confusion. The AI then understands your goal quickly and provides more accurate answers.
- Which prompt techniques are most helpful? Zero-shot, one-shot, few-shot, and chain-of-thought are popular. They let you adjust detail level, give examples, or reveal the AI’s reasoning.
- How do I control the AI’s creativity? Adjust the “temperature” setting. A low temperature (0.1) yields stable results; a higher one (0.8) creates more varied or inventive answers.
- Where should I start? Begin with short commands, test each prompt, and review the answers. Keep notes on what worked. Refine until you get the results you want.
Contents