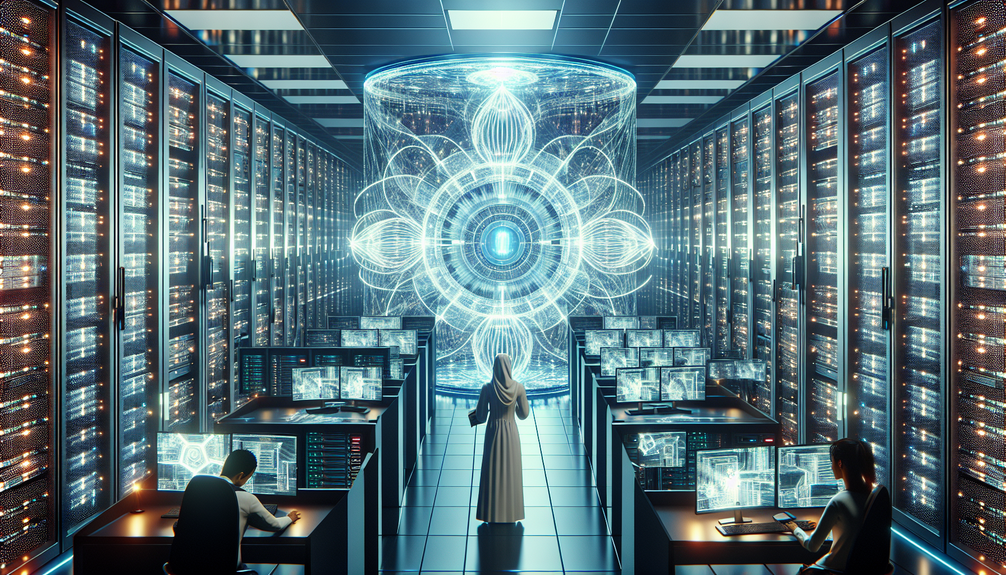
AI News
13 Apr 2025
Read 5 min
New MIT Method Protects Sensitive AI Training Data Efficiently
MIT’s new AI privacy method trains models faster, safer, and smarter—without slowing performance down.
MIT Researchers Develop a Faster and Safer Way to Protect AI Training Data
A team of researchers at MIT has created a new method to protect private or sensitive data used in AI training(data privacy). This method is faster and uses less computing power than current tools. It helps developers use personal or secret information without putting privacy at risk.
AI systems learn from large datasets. These datasets often include sensitive facts, such as medical records or financial information. If this data is not protected, it can be exposed, putting people’s privacy in danger. The new MIT method solves this problem using a smart defense strategy.
Why AI Training Data Needs Protection
AI systems are powerful because they learn from example. The better the examples, the smarter the AI gets. But many useful examples contain personal information. Today, many companies need strong tools to protect these datasets. If this data leaks, it can damage trust and even break legal rules.
Developers use a tool called “differential privacy” to protect data. However, differential privacy often slows down training and lowers accuracy. MIT’s new approach boosts privacy while keeping the AI fast, accurate, and secure.
How the New MIT Method Works
The MIT method protects data during the model’s training by adding small changes to the computer code that powers the AI.
Main Features Of MIT AI Training Data Privacy:
- Adds privacy without lowering performance
- Works faster than current tools
- Protects sensitive parts of the dataset
The method focuses on a weakness in current privacy tools. These tools treat all parts of the dataset the same way, but not all data is equally risky. MIT’s solution only adds the most protection where it is needed, saving time and computing power.
Saving Time and Energy in AI Training
Traditional privacy tools slow down training because they process all data the same way. MIT researchers made their method selective. It looks for parts of the data that pose a higher privacy risk and adds stronger protections only in those areas.
This makes the process faster and more energy-efficient. The system does not waste time fixing what isn’t broken. It focuses on the parts that need help, saving resources and delivering results sooner.
Real-World Uses for the MIT Method
This method can be helpful in many industries where privacy is key.
Examples:
- Hospitals can train AI to detect diseases without exposing patient records
- Banks can study customer behavior without revealing private transactions
- Government agencies can use AI to analyze data without risking leaks
It makes AI safer for fields where the wrong use of data can cause serious harm. Developers now have a tool that allows better use of this information without fear of data leaks.
A Smarter Way to Build Trust in AI
People worry about how their data is used. With this method, developers can train smart AI models while keeping personal data private. This helps build trust with users. When people know their data is safe, they are more willing to support and use AI tools.
Differential Privacy Made Lighter
Differential privacy works by hiding individual details in a dataset. It does this by adding random changes to the data. While this is a strong method, it can reduce how accurate the AI is. It also uses a lot of computing power.
MIT’s method keeps the strong parts of differential privacy but cuts out the waste. It adds just enough noise to protect sensitive parts of the data. This helps keep the model accurate without using extra resources.
Benefits Compared to Older Methods:
- Faster training times
- Better model accuracy
- Lower energy and computing needs
- Strong privacy protection where it matters most
Plans for Future Use
The MIT team hopes other researchers and companies will adopt this method. They believe it can become a new standard for training models with private data.
The goal is to support AI that is powerful, efficient, and trusted. More organizations now care about keeping data safe. This solution arrives at the right time for the AI field.
FAQ: MIT Protecting AI Training Data Privacy
1. What is the main idea behind MIT’s new method?
MIT’s method protects sensitive training data by securing only the most private parts of a dataset, which makes AI training faster and more accurate.
2. How is this different from standard differential privacy?
Traditional differential privacy treats all data the same, adding noise even to data that doesn’t need much protection. MIT’s way is smarter—it adds noise only where necessary.
3. What are the real-world uses of this method?
This method can help hospitals, banks, and government teams use AI tools without leaking private or sensitive information.
4. Will this method help AI models perform better?
Yes, by reducing computing needs and keeping important features in the data, the method can train better models faster without losing accuracy.
(Source: https://news.mit.edu/2025/new-method-efficiently-safeguards-sensitive-ai-training-data-0411)
For more news: Click Here
Contents