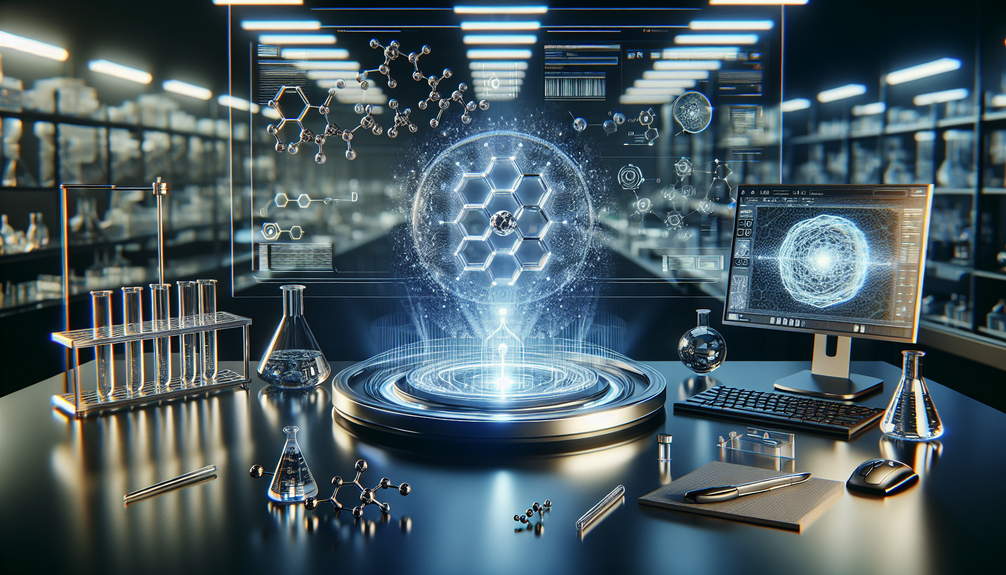
AI News
28 Nov 2024
Read 4 min
AI Revolutionizes Molecular Design with Fragment-Based Building Approach
AI revolutionizes molecular design by crafting molecules faster, cheaper, and smarter than ever before
How AI is Changing Molecular Design
Artificial intelligence (AI) is transforming many industries, and molecular design is no exception. Scientists now use AI to construct molecules one fragment at a time. This method has the potential to speed up the discovery of new materials and drugs. It also reduces the time and cost of traditional trial-and-error approaches used in chemistry. Welcome to the AI-driven molecular design!
The new AI tool uses a data-driven approach to learn how to design molecules. Instead of working with complete structures, it builds them step by step. Each step involves selecting a small chemical fragment and connecting it to others. This process mimics how chemists think but happens much faster and with fewer errors.
What is Fragment-Based Molecule Building?
Fragment-based building is a technique where smaller pieces, known as fragments, are used to construct larger molecules. These fragments can consist of atoms or small groups of atoms. Instead of guessing an entire molecular design, scientists start with basic components and combine them systematically.
This technique offers several advantages:
- It allows for a step-by-step process, making errors easier to catch and fix.
- It opens the door to discovering structures that might be overlooked in traditional methods.
- It uses fewer computational resources compared to analyzing entire molecules at once.
AI enhances this method by learning patterns and rules from vast amounts of data. The tool can predict which fragments will best connect and form stable, functional molecules. This speeds up the design process compared to manual or traditional computational methods.
How the AI-driven molecular design Tool Works
The AI tool is a type of generative model. It analyzes a molecular database to identify patterns among fragments. Then, it uses this knowledge to build new molecules.
Key steps include:
- Breaking down existing molecules in research databases into fragments.
- Learning how these fragments are commonly connected in functional molecules.
- Generating new molecule designs by combining fragments logically and efficiently.
- Testing output molecules for properties like stability and potential uses in materials or medicine.
This approach ensures that the molecules being designed are both innovative and practical.
Faster and Smarter Molecule Design with AI-driven molecular design
One major benefit of AI-driven design is speed. Traditional molecular design often requires extensive lab work and multiple failed attempts. AI eliminates some of this inefficiency by working through possibilities computationally before any lab work begins. This saves both time and money.
For example, the tool can help identify promising drug candidates or unique materials within hours, compared to weeks or months of lab testing. Researchers can focus directly on the most promising molecules.
Applications in Medicine and Beyond
Using AI to design molecules isn’t just useful for one field. It can be applied in several areas, such as:
- Pharmaceuticals: Discovering potential drug molecules with desired properties like solubility or target specificity.
- Materials science: Creating new materials for uses in electronics, energy storage, or construction.
- Environmental impact: Designing molecules to break down into harmless byproducts, reducing waste and pollution.
It’s important to note that AI doesn’t replace human chemists. Instead, it serves as a powerful tool to accelerate discoveries.
Challenges Still Ahead
While AI-driven fragment-based building is promising, there are still challenges:
- Data limitations: Predictions depend on the quality and quantity of data available to the AI.
- Complexity: Some molecules and reactions are too complex for current AI models to handle.
- Validation: AI can suggest molecules, but lab testing is still required to confirm key properties.
Researchers are actively working to improve these systems. Over time, they expect AI tools to become even more reliable and versatile.
What’s Next for AI in Chemistry?
AI advancements in molecular design are just the beginning. Experts believe fragment-based tools could integrate with other AI models that predict reactions or simulate properties. This would create an end-to-end solution for molecule creation, testing, and real-world application.
As more scientists adopt these tools, collaboration will play a significant role. Sharing data and improvements could lead to breakthroughs that push the boundaries of what is possible in chemistry.
Ultimately, AI has the potential to accelerate innovation far beyond the limits of human capabilities.
For more news: Click Here
Contents